Blog
The Power of Retrieval-Augmented Generation
RAG enhances AI text generation by combining retrieval with generation, improving accuracy and relevance in customer support, content creation, and research.
AI
Veröffentlicht am
13. November 2024
Aktualisiert am
13. November 2024

In the past few decades, artificial intelligence (AI) made tremendous advances in interpreting human language. Advanced models such as GPT and BERT arose; changing the way machines interpreted and responded to us, often sounding quite human. Despite their extraordinary powers, these models had significant drawbacks, such as being occasionally wrong or out of date. [1]
That is when the novel idea of Retrieval-Augmented Generation (RAG) came into play. This newer approach merged information retrieval with AI-generated responses, considerably improving the accuracy, relevance, and timeliness of results. [1]
In this post, let us dive into why Retrieval-Augmented Generation, or RAG, is shaking up how AI is used for creating content, supporting customers, powering research, and answering questions in real time. We will break down what makes RAG so effective and how it is transforming these areas for the better.
What is RAG?
First, let us take a moment to understand what RAG really is.
RAG, or Retrieval-Augmented Generation, is a hybrid AI framework that boosts the power of generative language models by combining them with a retrieval system. This means that, unlike traditional language models that rely only on their pre-trained knowledge, RAG can access and use external information from knowledge databases while generating responses. [2]
How RAG Works?
Now let us take a closer look at how RAG works, breaking down its key components and processes so we can fully understand its functionality and applications. RAG is a type of AI model that combines two powerful techniques: retrieval and generation.
Here is how it works:
Retrieval: When given a question or prompt, RAG searches a large database or set of documents to find relevant information. This step ensures the AI has access to accurate, up-to-date, and specific information rather than relying on just what it “knows” from training data. [1]
Generation: Once the relevant information is gathered, RAG uses a language model to generate a well-formed, natural response. This allows it to create responses that feel conversational and tailored to the user’s needs. [1]
By blending these two steps, RAG can generate responses that are not only highly accurate also feel dynamic and relevant.
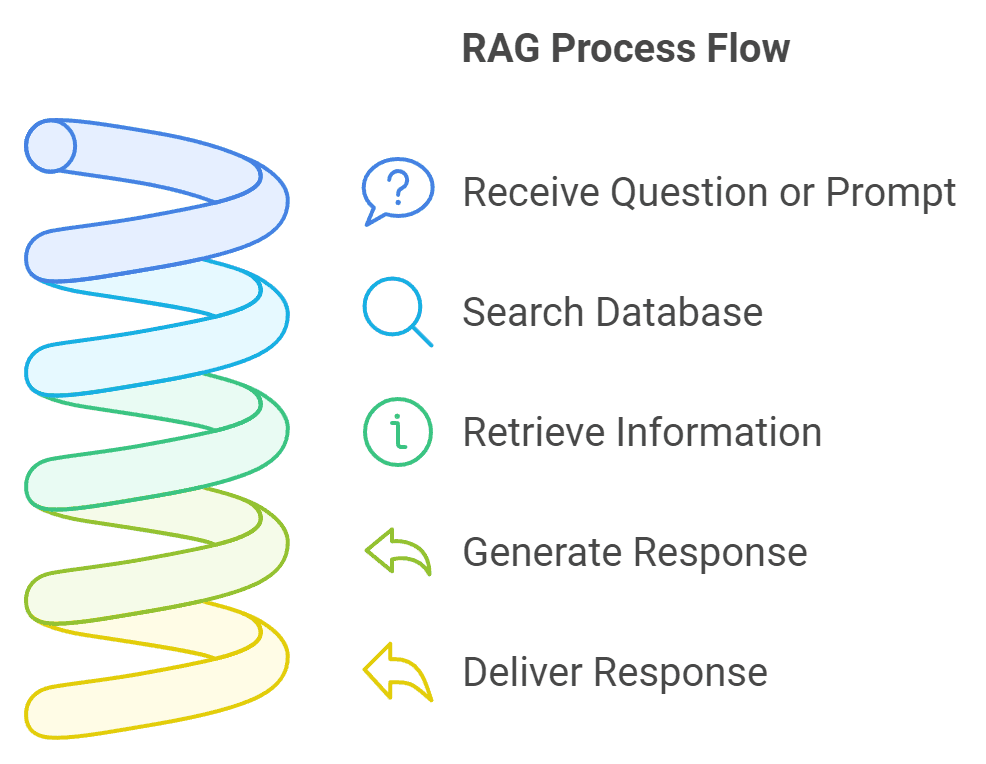
Why RAG is the Future of AI-Powered Text Generation?
Now, let us look at why RAG is set to redefine the future of AI. RAG stands out because it tackles some of the major weaknesses of traditional generative models. Here is why it is so innovative:
Trustworthy Factual Accuracy
A common issue with traditional AI models is their tendency to "hallucinate" facts, confidently presenting information that sounds correct but is actually false. This happens because these models rely solely on their pre-trained data and can sometimes miss the mark on accuracy. RAG, however, dramatically reduces these errors by sourcing information from reliable, trusted references. By integrating a retrieval system that accesses verified external data, RAG’s responses are not only more accurate but also more dependable. [3]
Access to Real-Time Knowledge
While most language models are limited to the static information they were trained on, RAG can access real-time information on the fly. This makes it perfect for situations that demand the latest data, whether it is recent news, cutting-edge research, or time-sensitive updates. Unlike traditional models, which can feel outdated as information evolves, RAG is built to deliver up-to-the-minute insights. [3]
Scalability across Various Industries
One of the greatest strengths of RAG is its adaptability. It is designed to gather knowledge from specialized databases, company resources, or even the open web, allowing it to cater to different industries and applications. This flexibility means that RAG can seamlessly adapt to roles in customer support, market research, healthcare, education, and more. Whatever the industry, RAG can retrieve context-specific information that makes it incredibly versatile and useful. [4]
RAG’s innovative approach not only improves factual accuracy and real-time access to information but also allows it to adapt to various industries. This makes RAG a powerful tool in the ever-evolving landscape of AI technology.
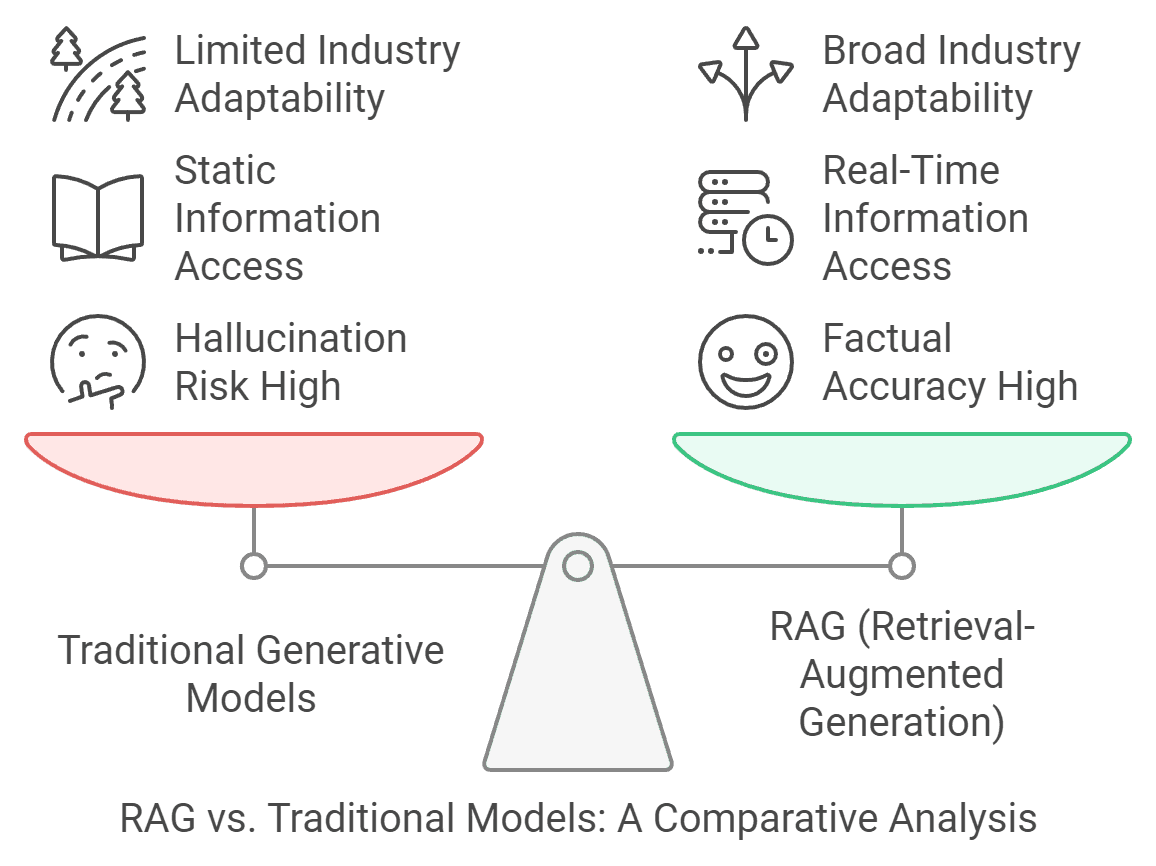
Applications of RAG
Now, let us dive into the real-world applications of RAG and see how it is making a difference across various fields. Imagine you are running a business or managing a research team, RAG can take your AI capabilities to a completely new level. Here is how:
Enhanced Customer Support: Imagine a chatbot or virtual assistant that can deliver answers with accuracy and speed, tapping into the latest information whenever it is needed. RAG-powered support systems do just that, significantly improving customer service operations. By incorporating RAG, businesses can ensure that customers receive responses that are not only accurate but also relevant and timely, leading to happier customers and more efficient support teams. [4]
Content Creation: For marketing and content teams, RAG is like having an assistant that is always up-to-date on the latest trends. It can help generate articles, product descriptions, social media updates, and more. This means content can be produced faster, with accurate, fresh information built right in. With RAG’s support, marketing teams can stay responsive to what is happening in their industries and produce content that resonates. [4]
Research and Analysis: In fields like market research, academic research, and competitive analysis, RAG shines by extracting insights from vast amounts of data. Whether it’s compiling the latest market trends, supporting academic research, or tracking competitor movements, RAG retrieves relevant information and generates comprehensive reports. This enables businesses and researchers to make informed, strategic decisions based on up-to-date knowledge and stay ahead of trends. [4]
In each of these areas, RAG’s ability to blend retrieval with generation is opening up exciting possibilities for organizations to work smarter and stay current.
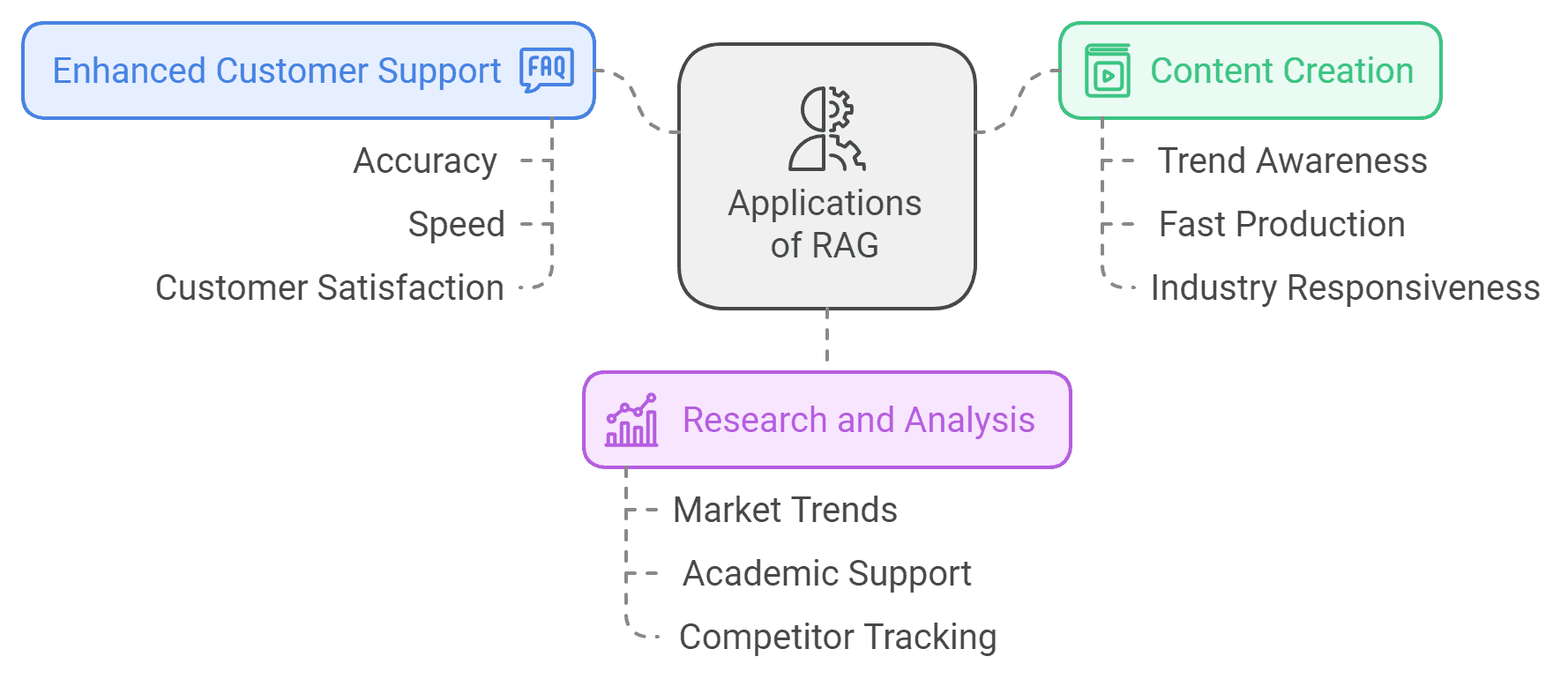
Benefits of RAG
Now that we have explored where RAG is making a real impact, let us look at the specific benefits that make it such a powerful tool. Here is how RAG goes beyond traditional AI models to deliver smarter, more accurate results:
Improved Accuracy: One of the biggest challenges with generative models is that they sometimes make up information, known as "hallucinations." By tapping into real-time, verified information, RAG significantly reduces these errors, providing responses that you can actually rely on. [2]
Real-Time Relevance: In a fast-paced world, having access to the latest data is essential. RAG’s ability to pull in up-to-date information ensures that its responses are not just accurate but also timely and relevant, making it perfect for applications that need the most current insights. [1]
Scalability: RAG’s flexible design allows it to be used across various industries, whether it is tapping into specialized knowledge bases or adapting to specific business needs. This makes RAG incredibly versatile, giving it the potential to support everything from healthcare and finance to education and beyond. [1]
Enhanced Contextual Understanding: By combining retrieval and generation, RAG can better understand the intent behind user queries, enabling it to respond in a way that is more contextually accurate. This means users get answers that are not just factual but also truly relevant to their questions. [2]
Trustworthiness: RAG does not just generate responses, it backs them up. By providing sources for the information it retrieves, RAG helps build user confidence, making its responses more credible and trustworthy. [6]
With these benefits, RAG is changing the game for AI, creating interactions that are smarter, more reliable, and better suited to today’s rapidly evolving information landscape.
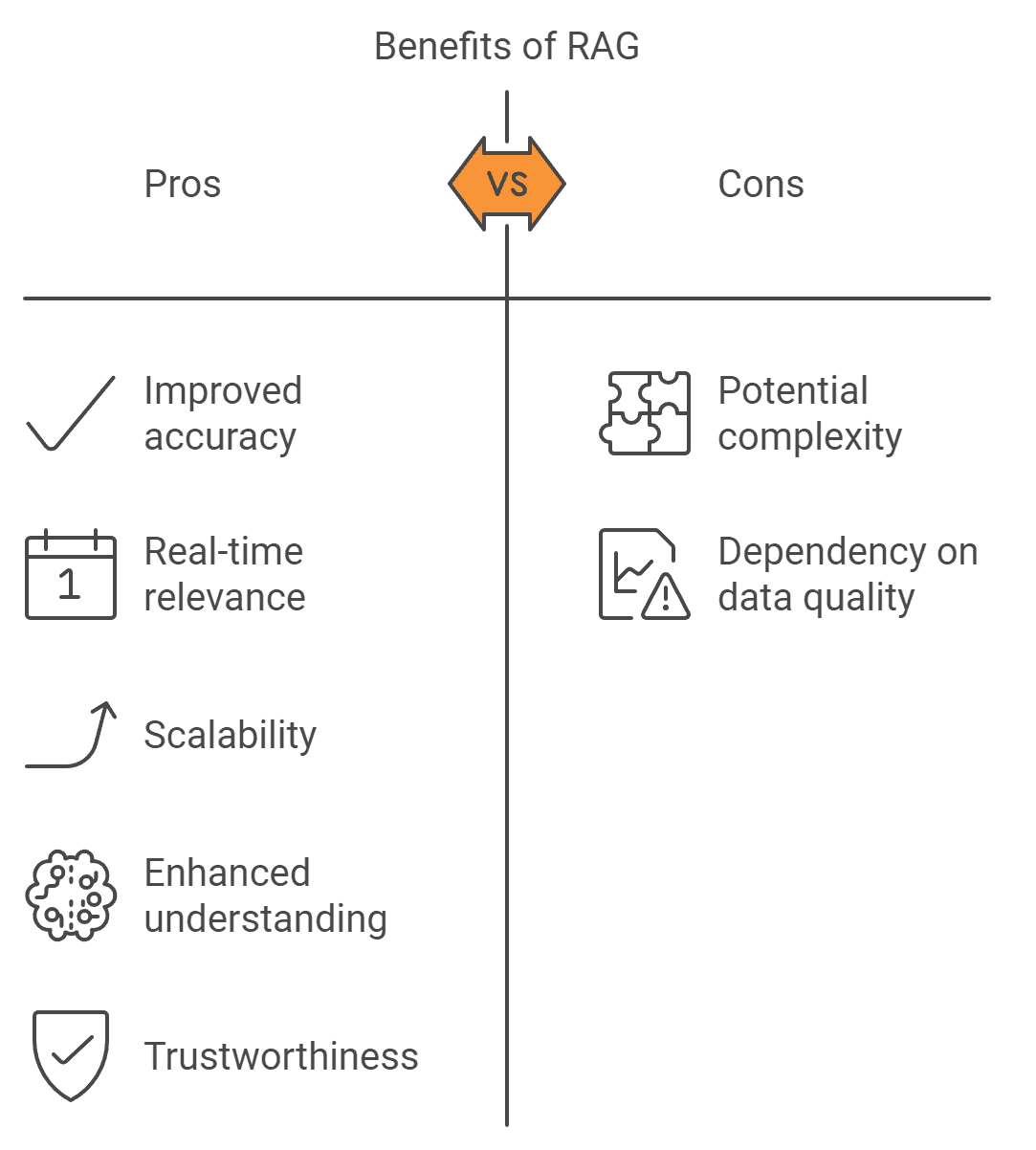
How RAG will change AI:
As we look to the future, it is clear that RAG will play a major role in the evolution of AI, shaping the next generation of intelligent applications. Here is a glimpse into what lies ahead with RAG:
A New Standard for AI Communication: RAG’s unique ability to blend real-world data with AI-generated responses brings us closer to AI that communicates with human-level accuracy and relevance. As the technology advances, we can expect even more precise and context-aware interactions, making AI a more natural part of our daily lives. [7]
Transformative Potential for Businesses: For businesses, RAG opens up exciting possibilities beyond simple automation. With access to real-time, factually correct, and contextually relevant information, AI-powered tools can deliver experiences that feel genuinely informed and responsive to each customer’s needs. [8]
Staying ahead in a Rapidly Changing Landscape: For companies looking to lead in the fast-moving world of AI, RAG represents the future. It is not just about generating impressive language; it is about grounding those responses in reliable, up-to-date knowledge. This blend of creativity and factual precision will be essential, as AI becomes a trusted resource in decision-making, customer support, and much more. [8]
RAG is not just enhancing AI; it is setting the stage for a future where AI interactions are richer, smarter, and more dependable than ever before.
Summary:
Retrieval-Augmented Generation (RAG) is a ground-breaking advancement in AI-powered text generation that enhances how machines provide information by merging retrieval systems with response generation. Unlike traditional models that often produce outdated or inaccurate replies, RAG searches for real-time AI knowledge from various databases before creating a response. This approach not only improves factual accuracy in AI but also ensures that answers are timely and relevant. RAG is particularly beneficial for applications in customer support, enabling chatbots and virtual assistants to deliver accurate and up-to-date responses. Additionally, it enhances content creation by helping marketers generate relevant articles and updates quickly. In the realm of research and analysis, RAG extracts insights from vast data sets, making it an invaluable tool for informed decision-making. By providing reliable sources for the information retrieved, RAG fosters user confidence and trustworthiness. As RAG continues to evolve, it promises to redefine AI interactions, setting a new standard for Natural Language Processing (NLP) that blends human-like relevance with factual precision in everyday applications.
Reference Links:
https://www.thinkingstack.ai/blog/case-studies-12/how-rag-ai-in-conversational-agents is-revolutionizing-customer-interactions-25
https://www.kommunicate.io/blog/rag-in-customer-service-chatbot/
https://dsmarinegroup.com/taking-advantage-of-the-power-of-retrieval-85/
https://blogs.nvidia.com/blog/what-is-retrieval-augmented-generation/
https://www.dataversity.net/rag-the-future-of-reliable-and-accurate-generative-ai/
https://www.upcoretech.com/insights/retrieval-augmented-generation-rag/